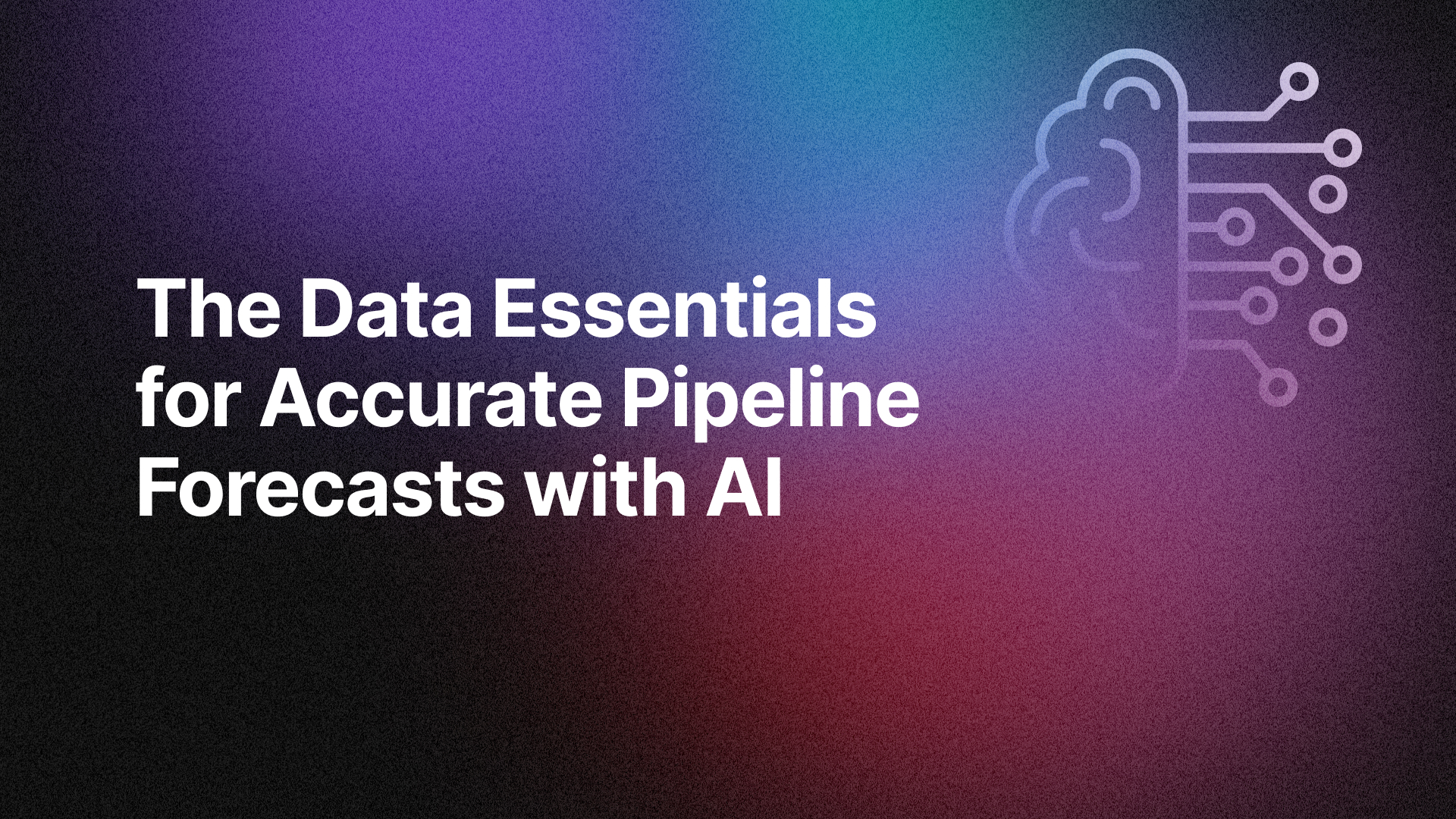
Accurate pipeline forecasting is essential for achieving revenue goals and making strategic business decisions. In B2B sales, relying solely on gut instincts or simple trend analysis is no longer enough. Today’s competitive landscape demands precise, AI-driven forecasts that provide a clear view of your revenue potential and pipeline health. However, even the most advanced AI models can only deliver reliable insights if they’re fueled by the right data.
To nail your pipeline forecasts, it’s crucial to establish a solid foundation, starting with clean and well-organized data. But data quality alone won’t provide the full picture. You’ll need to integrate key data sources—from CRM systems to marketing automation and Ad platforms—to ensure your AI model has a complete and comprehensive view of your pipeline. With the right approach to data hygiene, volume, weights, and a combination of forecasting methods, you can leverage AI to make accurate, data-driven pipeline projections.
1. Data Hygiene: Ensuring Clean and Reliable Inputs
Data hygiene is the cornerstone of accurate forecasting. Without high-quality, consistent data, even the best AI models will produce flawed predictions. Poor data hygiene introduces noise and errors, resulting in forecasts that can mislead teams and impact revenue planning. Ensuring your pipeline data is clean and reliable is the first step toward creating accurate AI-driven forecasts.
Key Aspects of Data Hygiene:
- Remove Duplicates: Duplicate records inflate your pipeline volume and skew win rates. Regularly audit your CRM to eliminate or merge duplicate entries, especially if multiple systems are feeding data into the pipeline.
- Consistency Across Systems: If your data comes from multiple sources, such as your CRM, marketing automation platform, and Ad, maintaining consistent naming conventions and formats is crucial. For example, the same company should not appear under different spellings (e.g., IBM vs. I.B.M.) in different systems.
- Timely Updates: To reflect the real-time state of your pipeline, data from each system must be up-to-date. Outdated information creates a false picture of where opportunities stand, particularly if their status has changed recently.
- Accurate Opportunity Data: Ensure that key data points—such as deal size, close probability, and expected close dates—are accurate. This data is critical for AI models to assess pipeline strength and generate precise revenue projections.
Without solid data hygiene, your forecasts are only as reliable as the cleanest part of your data. Ensuring that your CRM and other data sources are synchronized and free from errors is a foundational step for achieving precise AI-driven pipeline forecasts.
2. Data Volume: Feeding Your AI Model with Sufficient Historical Data
Volume is essential for AI models to generate accurate forecasts. The more historical data points your model can access, the better it can recognize patterns, detect trends, and project pipeline outcomes. However, achieving the right volume is not just about quantity; it’s about integrating relevant data from across your systems.
Why Volume and Integration Matter:
- Pattern Recognition: AI models can identify nuanced patterns across different customer segments, seasonal trends, and deal stages when they have access to a large data set. Pulling historical data from your CRM, Ad, and even customer success tools provides a holistic view that enables more accurate forecasting.
- Improved Predictive Accuracy: Larger data volumes allow AI to detect outliers, cyclical behavior, and other factors impacting pipeline health. With data from multiple systems, AI can account for variations in lead source, product line, and other characteristics to provide more accurate predictions.
For accurate pipeline forecasts, it’s critical to connect multiple data sources, including:
- CRM Systems (e.g., Salesforce, HubSpot): To provide core data on lead interactions, opportunity stages, and close probabilities.
- Marketing Automation Platforms (e.g., Marketo, Pardot): To add depth to the top of the funnel, offering insights into campaign-driven leads and marketing-sourced opportunities.
- Ad Systems: To add valuable data coming in from paid advertising platforms such as Google, and LinkedIn.
- Customer Success Platforms: To assess the likelihood of repeat business or upsells, enhancing long-term forecasting accuracy.
By connecting these data sources, your AI model gains a complete picture, helping improve accuracy in projecting both short-term and long-term revenue.
3. Weights: Assigning Value to Pipeline Data Points
Not all data points carry equal importance in pipeline forecasting. Weights refer to the relative importance assigned to each variable, ensuring that the most predictive factors contribute meaningfully to your forecast. By assigning appropriate weights, you can focus your model on the elements most likely to drive deal closure.
How to Determine Effective Weightages:
- Historical Performance Analysis: Reviewing historical data helps identify which factors correlate most strongly with deal success. For instance, if leads from a certain source convert at a higher rate, those leads should carry greater weight in your AI model.
- Engagement Scores: Weight recent, high-value engagement activities more heavily. For example, prospects who have recently attended webinars or scheduled demos should be prioritized over those who only visited a webpage.
- Behavioral Indicators: Weight behavioral indicators like deal velocity, recent interactions, and frequency of engagement. Prospects with recent activity may indicate higher buying intent, justifying higher weightage in the forecast.
To establish weights, AI models need consistent data from CRM and marketing automation platforms, ensuring that engagement data and deal characteristics are factored inaccurately.
4. Integrating Forecasting Methods for Comprehensive Pipeline Predictions
The most accurate pipeline forecasts use a blend of forecasting models. No single approach captures every aspect of the pipeline, so combining different methods provides a fuller picture.
Key Forecasting Methods to Consider:
- Time Series Analysis: Analyzes historical revenue and pipeline data to detect patterns and project future outcomes. Connecting to CRM and Ad data ensures that time series analysis accounts for trends across the customer lifecycle.
- Regression Analysis: This approach assesses how variables like deal size, lead source, and industry impact revenue outcomes. Pulling data from CRM and marketing platforms enables regression models to predict which leads are likely to convert based on these attributes.
- Classification and Clustering: By categorizing leads and opportunities based on characteristics, classification models help prioritize deals with the highest potential, while clustering models uncover hidden segments.
- Scenario Modeling: Tests different outcomes based on market changes or sales adjustments. Scenario modeling uses CRM and Ad data to evaluate best- and worst-case revenue projections.
Making Pipeline Forecasting Data-Driven and Precise
RevSure’s Pipeline Projections Module combines these methods to provide a multifaceted view, pulling data from multiple sources for the most accurate pipeline forecasts. It brings your pipeline forecast to life by integrating key data from CRM, marketing, and Ad systems, ensuring a complete and accurate picture of your pipeline’s future. RevSure enables data-driven, precise predictions for more effective pipeline management.
Ready to turn your pipeline into a predictive powerhouse? Book a demo with RevSure today to see how our Pipeline Projections Module can transform your pipeline forecasting with data-driven precision.