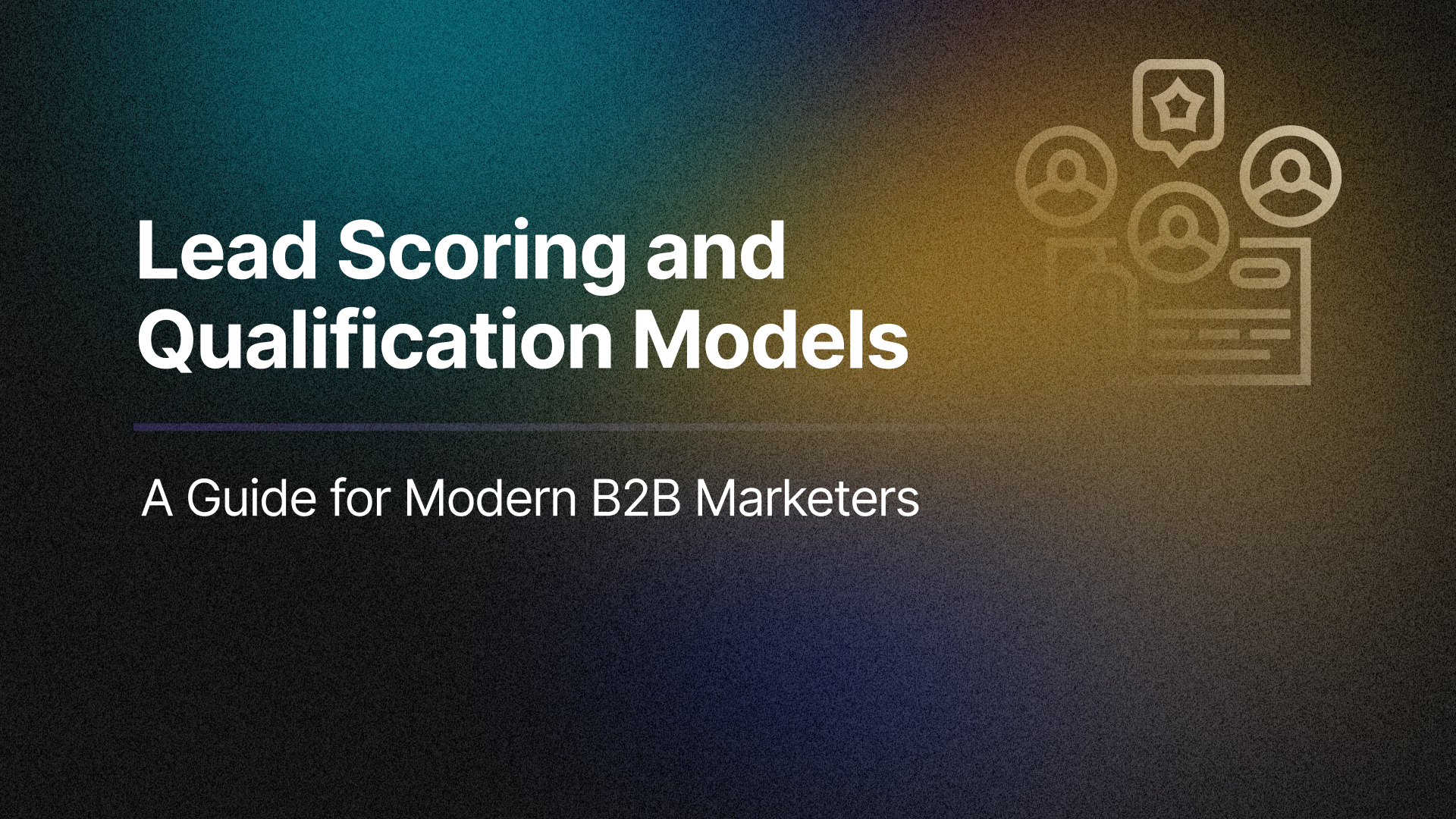
Lead scoring has grown beyond simplicity, becoming critical in aligning sales and marketing teams. More than relying solely on traditional models to categorize leads into buckets like Marketing Qualified Leads (MQLs), Marketing Engaged Leads (MELs), or Sales Qualified Leads (SQLs) is required to meet the demands of modern B2B strategies.
The modern lead-scoring approach must incorporate stochastic methods, leveraging firmographics, behavioral data, and intent signals to create a nuanced predictive lead-scoring system. This article explores the common pitfalls in lead scoring, shares proven strategies to refine your approach, and highlights methods for building lead qualification models that deliver value in a fast-changing market.
Understanding Real vs. Synthetic Events in Lead Scoring
It's important to distinguish between real and synthetic events to build an effective lead-scoring model:
- Real Events: These are tangible actions that have occurred, such as a discovery call. These provide precise, actionable data reflecting direct engagement between the prospect and your company.
- Synthetic Events: These are primarily composite actions coming from multiple signals. For example, an MQL or SQL status often results from a combination of behaviors—such as attending a webinar, downloading content, or interacting with your website. While synthetic events capture broader patterns of intent, they are subjective and require sophisticated models to assess their true value.
In a modern world lead-scoring framework, you will need a mix of real and synthetic events. Real events will lay the ground for scoring, while synthetic events provide a complete view of prospect engagement and interest.
Best Practices for Effective Lead Scoring Models
To build a robust and reliable lead-scoring model, consider the following best practices:
Combine Real and Synthetic Events
Lead scores should account for tangible actions (discovery calls, demo requests) and aggregated behaviors (webinar attendance, multiple downloads). This composite approach provides a complete picture of an account or a lead's engagement and purchase readiness.
Use Stochastic, Not Deterministic Scoring
Deterministic scoring assigns fixed values to specific actions, such as +10 points for an email open or +50 points for a demo request. This is a very simplistic approach and will need more nuance. A stochastic scoring model uses probabilities and weights to account for variability, recognizing that similar MQLs might behave differently depending on firmographics, intent signals, and buying stages.
For example:
- A demo request from an ICP might receive a higher score than a non-ICP lead with the same action.
- Intent signals from external sources, like 3rd party research, could further refine the weights.
Incorporate Intent Signals and Firmographics
To make lead scores more predictive:
- Align scores with your Ideal Customer Profile (ICP), incorporating firmographics such as industry, company size, and geography.
- Augment lead data with intent signals show interest in specific solutions or topics. These signals can be captured from third-party platforms or first-party analytics, providing insights into the lead's buying journey.
Validate Models with Regression Testing
Regularly test lead scoring models against historical data to validate their effectiveness. Use regression testing to:
- Identify patterns and refine scoring weights.
- Measure how well the model predicts conversion rates and pipeline.
- Adjust for changes in market conditions or customer behavior.
Collaborate Across Different Teams
Lead scoring is not just marketing overhead anymore—it also requires collaboration with sales and operations teams. Regularly review scoring models with these teams to ensure alignment and identify areas for improvement. For example:
Keep Models Adaptive
Market conditions and buyer behavior are constantly changing. Ensure that lead scoring models are flexible and relevant with new data flowing in and evolving business requirements. Incorporate ML models that can learn from past conversions and adjust scores dynamically.
Challenges in Lead Scoring and Qualification
While lead scoring offers significant advantages, it comes with its own set of challenges:
Complexity of Scoring Models
Modern lead-scoring models require extensive data inputs, including engagement metrics, firmographics, and intent signals. Balancing simplicity and sophistication is key:
- More complex models may need to be clarified for stakeholders and reduce adoption.
- Simplistic models must pay more attention to critical nuances impacting lead quality.
Data Requirements
Accurate lead scoring relies on high-quality data. Key challenges include:
- Ensuring enough data for model training and validation is always a challenge.
- Avoiding gaps or inconsistencies within data that skew results.
- Minimizing reliance on costly, sometimes inaccurate data enrichment.
Costs vs. Insights
While data enrichment enhances lead scoring, it can also inflate costs. Marketers must balance the expense of acquiring enriched data with the value it adds to the scoring model:
- Focus on high-impact enrichment, such as adding firmographic and intent data for ICP-aligned accounts.
- Regularly audit data sources to ensure cost-effectiveness.
The Role of ML in Lead Scoring
Here's how ML can enhance lead scoring:
1. Predictive Scoring: ML models can analyze historical data to identify conversion patterns. Based on these insights, scores can be assigned dynamically, improving the accuracy of lead prioritization.
2. Continuous Optimization
ML-based systems learn from new data and adjust scores over time, ensuring that lead scores remain relevant as buyer behavior evolves.
3. Improved Segmentation
ML enables granular segmentation by identifying patterns within the data, helping marketers differentiate between leads. With improved segmentation, GTM teams can prioritize those with the highest conversion potential.
Customized Event-Based Scoring with RevSure
With RevSure's customizable Lead Scoring Model, you can derive scores based on specific events to evaluate leads effectively as they progress through the funnel. This capability allows you to:
- Assign scores when a lead advances stages, opens an email, reads your contract, or watches your video.
- Customize scoring to align with the significance of each activity in driving conversions.
- Score actions that matter most to your sales process to better understand lead intent and readiness to convert.
Conclusion
Lead scoring and qualification models are critical for driving B2B marketing and sales efficiency. Marketers can create more nuanced and predictive systems by incorporating real and synthetic events, leveraging stochastic scoring methods, and integrating firmographics and intent signals.
In the current B2B landscape, the ability to prioritize high-potential leads with precision can make the difference between meeting or missing your revenue goals.
Download our "Best Practices for Marketing Automation Setup & Attribution" ebook for more insights.
-p-2600.jpeg)