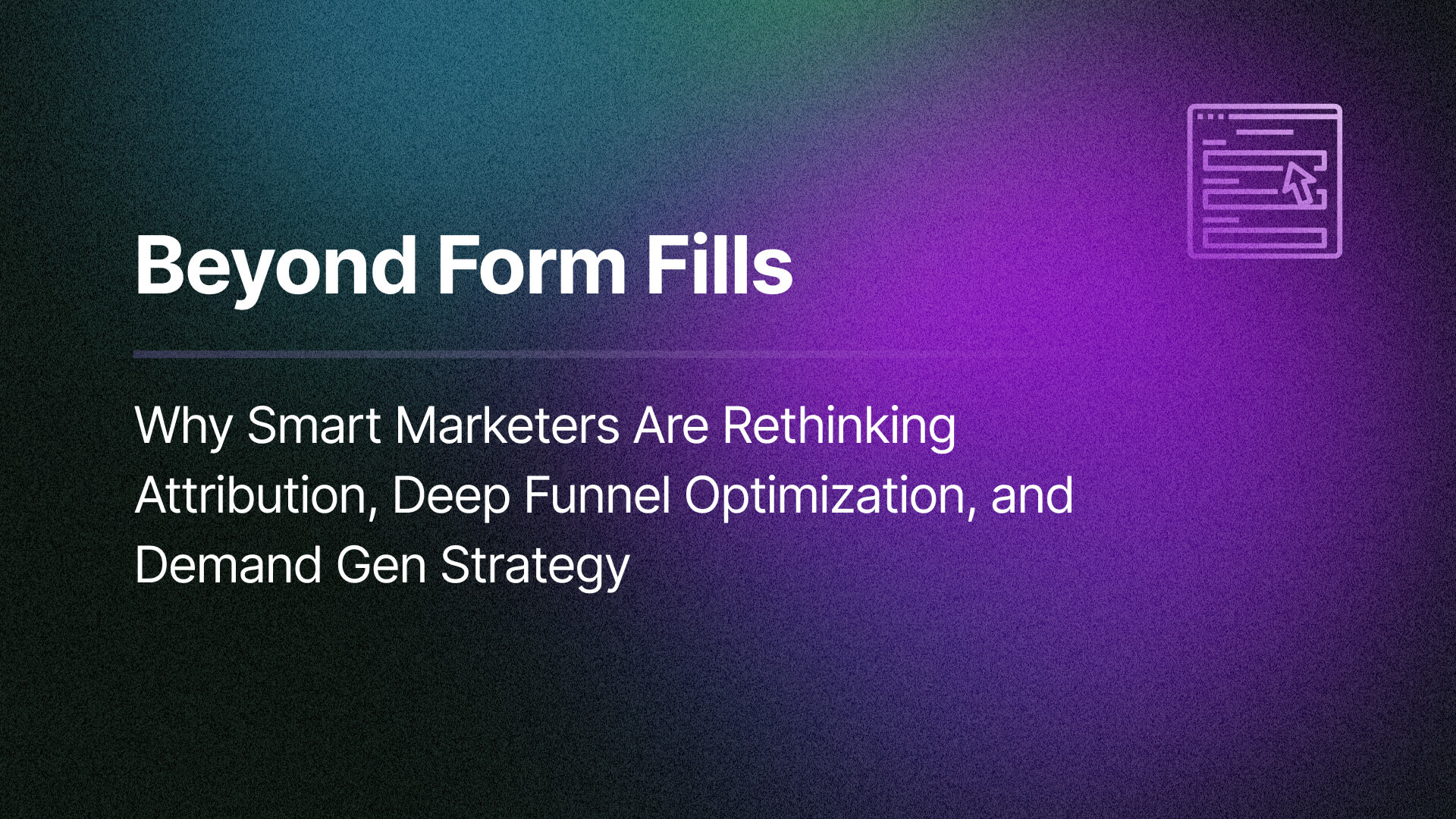
In the age of data-rich marketing and AI-powered execution, we’ve reached a pivotal moment: traditional lead generation models and attribution frameworks are no longer enough. The buying journey is longer, messier, and more complex than ever—and the stakes for profitable growth are rising.
To keep pace, leading marketers are going beyond basic campaign tracking and into the realm of deep funnel optimization, influence-based attribution, and statistical lift testing. This isn’t just a shift in tactics—it’s a shift in mindset.
Let’s explore why modern marketing teams are re-engineering their approach from the ground up, and how these next-gen methods redefine what high-performance B2B marketing looks like.
Attribution Isn’t Broken—Your Model Might Be
For years, attribution was treated as a math problem: divide credit among touchpoints, pick a model (first, last, or multi-touch), and use that to justify spend. But in reality, attribution is not about who gets credit—it’s about uncovering influence.
When you examine real buyer journeys, especially in mid-market and enterprise deals, the flaws of simplistic models become obvious. Deals often involve thousands of touchpoints over months or even years—email opens, webinar registrations, social media engagements, trade show scans, nurture sequences, and more.
Pinning a seven-figure deal to a single first or last click is reductive—and dangerously misleading. Yet, many marketers continue to lean on these metrics simply because they’re easy to report.
That’s where influence-based attribution enters the picture. Instead of selecting one touchpoint to credit, influence models analyze all interactions to understand how each contributed to forward momentum in the funnel. Think of it less like slicing a pie and more like mapping a constellation—uncovering the relationships between actions and outcomes.
Smart marketers aren’t asking “Who gets the credit?” anymore. They’re asking:
- Which touchpoints consistently influence deals that convert?
- Which combinations of actions lead to velocity in the pipeline?
- What motions repeat in successful deals, and how can we double down on them?
The Rise of Lift Testing and Statistical Rigor in Marketing
Today’s marketing teams don’t just need attribution—they need proof. This is where lift testing and incrementality analysis come in. Instead of guessing which campaigns “probably worked,” marketers are adopting controlled test frameworks that evaluate whether a campaign or channel truly impacted conversion rates or pipeline creation.
Lift tests allow you to isolate a cohort—those who were exposed to a campaign or activity—and compare outcomes against a control group. The goal isn’t to win a debate about attribution; it’s to learn what actually moves the needle.
For instance:
- Did your paid search campaign drive incremental pipeline?
- Did your webinar audience show statistically higher conversion?
- Are your retargeting ads lifting MQL-to-SQL rates, or are they just cannibalizing organic intent?
If your marketing strategy doesn’t include statistical confidence alongside performance metrics, you’re still flying blind. And, the harsh truth is: most B2B marketers weren’t trained as data scientists. That’s why pairing AI with marketing analytics has become a game-changer.
Deep Funnel Optimization: Your Untapped Growth Lever
It’s time to stop optimizing for cheap leads—and start optimizing for valuable conversions. Deep Funnel Optimization is a framework that focuses on the full buyer journey, from the initial conversion all the way through to closed-won. Rather than judging performance solely on lead quantity or cost-per-click, marketers using deep funnel thinking focus on signals that predict downstream outcomes—like pipeline creation, sales velocity, and customer acquisition cost (CAC).
Here’s the reality: platforms like Google and Meta are designed to optimize for whatever signal you give them. If you tell them the goal is form fills, they’ll find users most likely to fill out forms—even if those people are completely unqualified.
But if you pass back post-lead signals like MQL, SQL, opportunity creation, or even Gong call participation, the ad platforms will optimize toward those behaviors instead.
And the payoff is significant. Teams using Deep Funnel Optimization frequently report:
- 35%–50% lower CAC
- Shorter sales cycles
- Higher lead-to-opportunity conversion rates
- Improved alignment between sales and marketing
It also eliminates a massive pain point: the volume trap of sending sales hundreds of low-quality leads and eroding trust between teams.
Why Synthetic Events Are Critical for Optimization
In B2B, deals don’t close in seven days. That’s a problem when ad platforms like Meta only give you a short window to attribute success. That’s why leading marketers now manufacture synthetic events—meaningful milestones that happen post-form fill but pre-close. These include:
- Booking a discovery call
- Attending a webinar
- Receiving a sales sequence via Outreach
- Getting a high-fit score from 6sense
- Having a 30+ minute call recorded in Gong
Each of these becomes a proxy signal of engagement and buying intent. By piping these signals back into Google or Meta, marketers train the algorithms to prioritize users who act like buyers, not just clickers.
The magic happens when you pair these synthetic events with dynamic value scoring—giving each event a weighted value based on its likelihood of resulting in revenue. This isn’t deterministic “every MQL is worth $100” logic. It’s probabilistic modeling using fit, behavior, and funnel velocity.
Think of it as building your own “truth set” to teach platforms what good looks like.
Signal Density > Signal Volume
Sending a massive number of signals to ad platforms may sound appealing, but it’s not about volume—it’s about precision. The most effective demand gen teams send varied, high-quality signals that capture the nuances of their buyer journeys. These teams understand:
- How to calibrate values across lead scores, funnel stages, and segment fit
- When to retract bad signals (e.g. spam leads or bot clicks)
- How to create differentiated models for enterprise vs. SMB motions
- Why more expensive leads can actually improve CAC, not hurt it
The goal is not to reduce cost per lead—it’s to reduce cost per pipeline and improve deal efficiency.
Operationalizing AI for Real Impact (Not Just Content Creation)
Much of the AI buzz in marketing has centered on generative content tools. But the real transformative value lies in AI-driven decision-making and optimization.
AI is already driving:
- Attribution modeling based on touchpoint influence and deal outcomes
- Conversion lift prediction based on behavioral and firmographic signals
- Spend optimization across high-ROI channels
- Dynamic scoring of leads and opportunities
It’s not about replacing marketers—it’s about enabling them to focus on strategy while AI handles the statistical heavy lifting. And importantly, it's about creating a flywheel where every signal fed into AI models improves future outcomes, reduces inefficiencies, and scales what works. Check out this video in conversation with Harry Hawk, where advanced conversion signals, AI-powered attribution, and deep funnel optimization are discussed. If you're looking to cut CAC, shorten deal cycles, and make your paid spend work harder—this one's for you.
From Lead Gen to Pipeline Intelligence
The most forward-thinking marketing teams have already started evolving from “demand generation” to pipeline engineering.
That means:
- Moving away from vanity MQL goals
- Partnering with sales and customer success on shared metrics
- Re-architecting tech stacks to track meaningful touchpoints across the full journey
- Using attribution not for credit—but for learning, iteration, and growth
They’re no longer asking: How many leads did we get this month?
They’re asking: How many opportunities did we influence? What worked—and how do we replicate it?
This is the future of B2B marketing—and it’s already here.
Final Thought: Complexity Isn’t a Bug—It’s the System
B2B marketing today is nuanced, multi-layered, and hard to measure. That’s not a failure of the system—that is the system. Top marketers aren’t fighting the complexity. They’re building systems to embrace it—layering AI models, statistical frameworks, and deep funnel insights to make smarter decisions, faster.
If your team still operates with simplified attribution models and surface-level metrics, you’re not just leaving money on the table—you’re ceding competitive advantage. The future belongs to marketers who think like engineers, measure like analysts, and execute like strategists. Ready to upgrade?