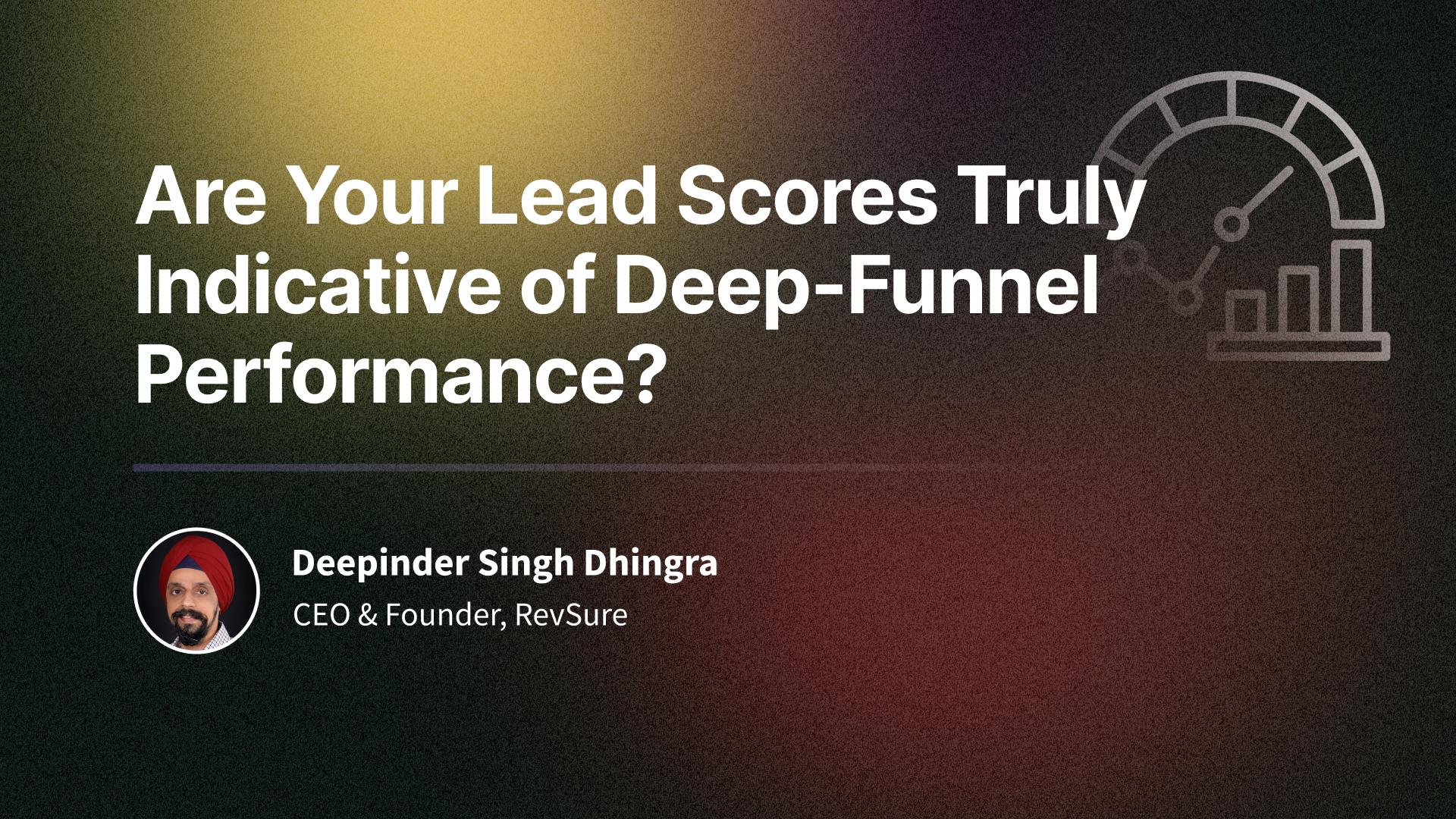
How often have we heard from marketing leaders that MQLs (Marketing Qualified Leads) are being overlooked by SDR (Sales Development Representatives) and BDR (Business Development Representatives) teams?
Despite enhancements in lead scoring systems, many sales teams remain skeptical of lead scores as indicators of real pipeline potential. The reality is that while lead scoring has become increasingly sophisticated, moving beyond simple engagement metrics to include intent and digital activities, many B2B SaaS companies still find that these scores don’t reliably predict conversion to pipeline or closed deals.
So, if today’s lead-scoring methods aren’t effectively moving the needle on conversions, why is that? Let’s explore why lead scores often fall short and what can be done to bridge the gap.
The Evolution of Lead Scoring—and Its Limitations
Lead scoring was initially designed to help sales teams prioritize outreach by assigning a numerical score to each lead based on factors like fit (e.g., industry, company size) and engagement (e.g., email opens, and website visits).
With advances in data tracking, today’s lead scores can also incorporate buyer intent signals, social media interactions, form-fills, social media post interactions, impressions, and other digital activities. This deeper level of scoring theoretically offers a richer view of lead readiness.
However, despite these advancements, many SDR and BDR teams are still wary of relying on lead scores alone. They’ve experienced cases where leads with high scores don’t actually convert to opportunities at higher rates, leading them to question the effectiveness of the scoring system.
In fact, our recent analysis of 21,000 MQLs from a B2B SaaS company showed that as the lead score increased from a “D” rating to an “A” rating, the conversion rate to the pipeline actually dropped. Instead of correlating positively with conversions, higher lead scores were negatively correlated to pipeline performance.
Why Are High Lead Scores Not Converting?
So, what’s going wrong? Why are leads with high scores not converting as expected? Here are the main reasons lead scores often fail to predict deep-funnel performance:
1. Rules-Based Scoring with No Feedback Loop
Most lead-scoring models are rules-based. They assign scores based on predefined criteria—whether that’s a form-fill, a visit to a pricing page, or multiple email opens. However, these scores don’t take into account how these leads are actually converting (or not) further down the funnel. There’s no feedback loop that ties actual conversion data back into the scoring model.
Without a feedback loop, the scoring model can’t “learn” from past performance. If certain behaviors or attributes consistently lead to conversions, these should influence future scores. Unfortunately, many lead scoring systems don’t have this capability, which makes them blind to what really drives conversions over time.
2. Failure to Capture the Right Signals
Even with advanced tracking, lead scores often fail to capture the right signals indicative of true buying intent. In many cases, lead scores are built around top-of-funnel activities, like initial website visits, form-fills, or ebook downloads. While these actions might show initial interest, they don’t necessarily indicate a readiness to engage with sales or enter the buying process.
High-converting signals, such as revisiting a pricing page, viewing a specific case study, or returning to the product section multiple times, often carry more weight. Unfortunately, many lead scoring models still lack the granularity needed to weigh these behaviors accurately, resulting in scores that aren’t predictive of genuine sales potential.
3. Focus on Top-of-Funnel Metrics and Lack of Alignment with Sales Dynamics
Lead scores are often based on top-of-funnel metrics, such as engagement and fit, rather than mid- to bottom-funnel dynamics. They don’t account for the nuanced, interactive journey that takes place between SDRs/BDRs and leads further down the funnel. For example, if an SDR has multiple positive interactions with a lead, or if an Account Executive (AE) observes consistent interest from a lead at a specific buying stage, this engagement isn’t factored into most lead scoring models.
Lead scores also don’t account for how sales conversations might impact lead readiness. A lead could initially score high due to digital interactions but may have shown hesitation or disinterest in subsequent calls with sales. Since traditional lead scoring models often ignore these live insights from the SDR/BDR and AE motions, they miss the opportunity to adapt to the actual conversion potential.
What Needs to Change? Towards a Predictive, Feedback-Based Approach
To make lead scoring more predictive of pipeline and revenue outcomes, B2B marketers and sales teams need to move away from rigid, rules-based models and embrace a data-driven, feedback-oriented approach. Here’s how:
1. Integrate Conversion Feedback Loops
A feedback loop that incorporates data from closed deals back into the scoring model would allow it to “learn” from conversion behavior. By analyzing which attributes and interactions truly correlate with conversions over time, the model could adjust its scores to better reflect genuine buying intent. This would create a more predictive system that gets smarter as more data flows through it.
2. Prioritize High-Intent Signals Over Surface-Level Engagements
Rather than focusing primarily on top-of-funnel activities, the scoring model should prioritize high-intent behaviors that signal conversion potential. For example, a return visit to the pricing page, a demo request, or a high level of content engagement around product-related topics could be weighted more heavily than one-off interactions. This would help the model distinguish between leads that are casually browsing and those with genuine purchase intent.
3. Align Scoring with Sales Insights and Dynamics
For lead scoring to drive real pipeline value, it needs to consider sales dynamics and mid to bottom-funnel interactions. Instead of only scoring based on static engagement metrics, models should incorporate insights from sales conversations and feedback from SDR/BDR teams. By taking into account how leads respond in actual sales interactions, scoring models can better reflect the nuances of the buyer journey and better align with sales priorities.
A New Approach: Predictive Intelligence for Lead Scoring
At the end of the day, modern lead scoring requires a predictive, data-based approach rather than one that simply assigns points based on arbitrary rules. Predictive intelligence and AI-driven models can analyze thousands of data points to identify patterns and correlations between engagement behaviors and conversions. By using machine learning to analyze historical conversion data, predictive scoring models can continuously refine their scoring criteria to align with actual sales outcomes.
This approach is also dynamic, updating in real-time as new engagement data becomes available. It provides SDR/BDR teams with up-to-date, reliable insights into which leads are genuinely ready for conversion, reducing skepticism and fostering stronger alignment between marketing and sales.
Conclusion
In B2B marketing, lead scoring can be a powerful tool for prioritizing leads, but only if it truly reflects conversion potential. Traditional, rules-based scoring systems often fail to predict pipeline outcomes because they lack a feedback loop, ignore high-intent signals, and don’t align with the sales process. By embracing a predictive, data-driven approach that learns from actual conversions, B2B companies can turn lead scoring into a reliable driver of pipeline growth.
If your lead scores aren’t delivering the results you need, consider evolving your approach. Incorporate feedback loops, focus on high-intent signals, and align scoring with sales insights to create a lead scoring model that is truly indicative of deep-funnel performance.