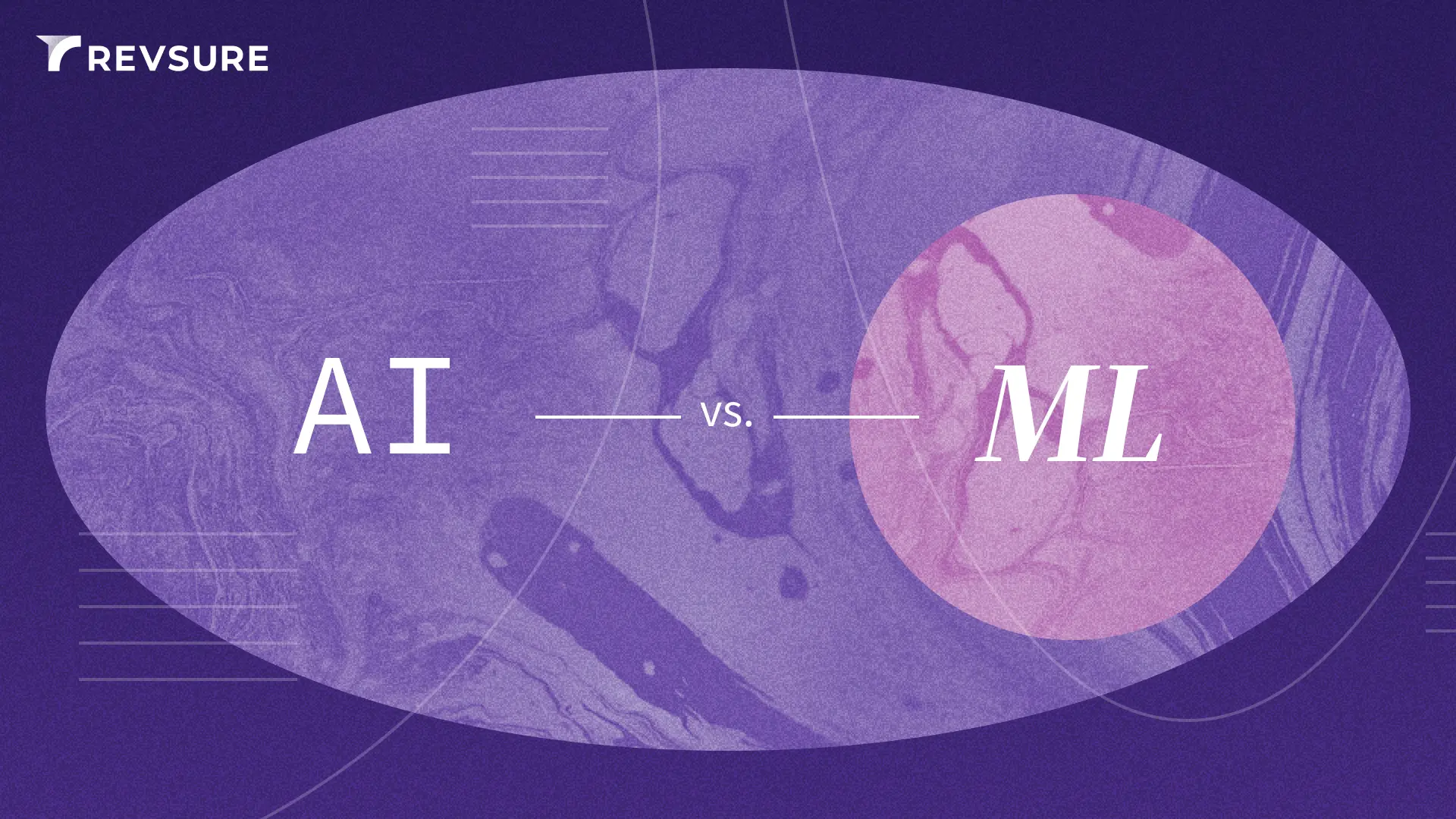
While everyone is talking about the AI trend now, did you know it’s actually been around since the mid-1950s? The term "artificial intelligence" was coined by John McCarthy, who is considered one of the founding fathers of AI. Early work in AI focused on developing symbolic or rule-based systems that aimed to mimic human intelligence. These systems used logical rules and knowledge representation techniques to solve problems and make decisions. However, progress was limited due to the lack of computational power and the complexity of modeling human intelligence.
Today, AI is a rapidly evolving field with diverse applications, including natural language processing, computer vision, robotics, autonomous vehicles, recommendation systems, and more. The development and deployment of AI technologies continue to shape various aspects of our lives and industries.
One thing that isn’t so clear to everyone – what’s the difference between AI (Artificial Intelligence) and ML (Machine Learning)? And what is AI–outside of ChatGPT?
The Difference Between AI and ML
Artificial Intelligence (AI): AI is a broad field that encompasses the development of intelligent systems capable of performing tasks that typically require human intelligence. It focuses on creating machines that can simulate human thinking and decision-making processes. AI aims to build systems that exhibit behaviors like learning, reasoning, problem-solving, perception, and natural language processing.
Some examples of AI include virtual assistants like Siri or Alexa, autonomous vehicles, and image or object recognition.
Machine Learning (ML): ML is a subset or subfield of AI that focuses on the development of algorithms and statistical models that enable computers to learn from and make predictions or decisions based on data. ML algorithms are designed to automatically analyze and learn patterns from large datasets without being explicitly programmed. ML models can improve their performance over time as they are exposed to more data, allowing them to make accurate predictions or decisions in specific tasks.
Some examples of ML include predictive analytics, fraud deduction, and spam filtering in your inbox or cellphone.
In essence, ML is a technique used within the broader field of AI. ML algorithms are a means to achieve AI by enabling machines to learn and make intelligent predictions or decisions. AI, on the other hand, encompasses a broader range of concepts and approaches beyond just ML, including rule-based systems, expert systems, knowledge representation, and more.
Types of Artificial Intelligence
There are several types of AI, natural language processing, reinforcement learning, machine learning, etc. But we want to drill in on the differences between generative and contextual.
Generative AI: Generative AI refers to a class of AI models that are designed to generate new content or data that resembles the input data they were trained on. These models learn the underlying patterns and structures of the training data and can generate new samples based on that learned knowledge. Generative AI models can be used for tasks such as image synthesis, text generation, music composition, and more. They can produce new and original content, often with some degree of creativity.
A few examples include ChatGPT, creative imagery platforms, or a photoshoot from selfies.
Contextual AI: Contextual AI, on the other hand, focuses on understanding and processing information within a given context. It aims to interpret and respond to data based on the specific context in which it is presented. Contextual AI models take into account various contextual factors, such as the environment, user preferences, historical data, and other relevant information to make informed decisions or provide appropriate responses. Contextual AI is often used in applications like natural language processing, chatbots, recommendation systems, and personalized user experiences.
Examples of contextual AI include personalized recommendations on Netflix, virtual assistants, and customer support chats.
So, while some think AI is new and a trend that may play out, it’s been around for decades and is only growing in scope and popularity. While others think it will take over the world, many are embracing it to become more efficient in their daily lives and at work. What will you choose?