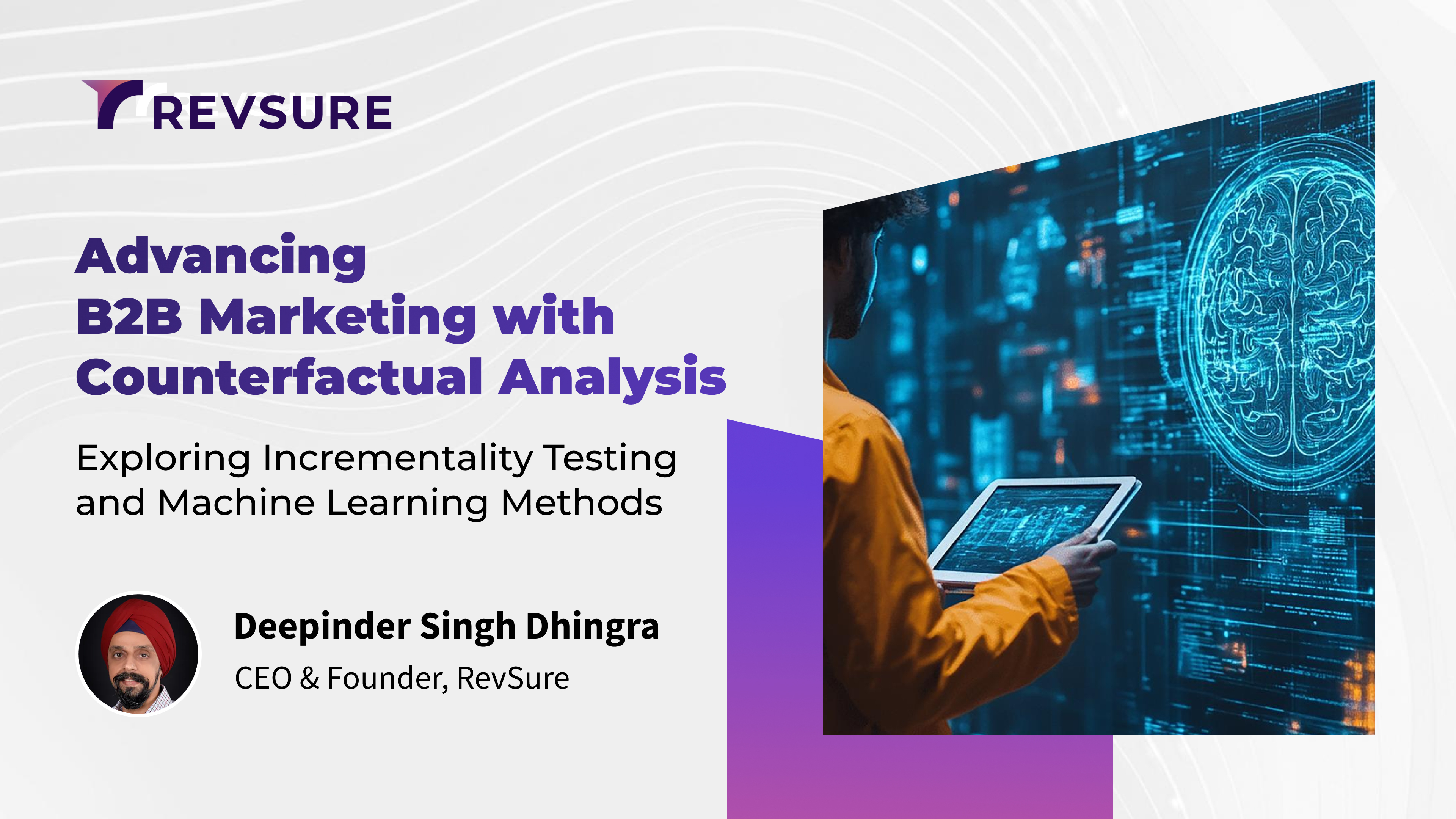
In a previous article, we discussed the importance of counterfactual thinking in B2B marketing and its various applications. Counterfactual thinking allows marketers to envision alternative scenarios and better understand the true impact of their strategies. Building on that foundation, this article will explore two specific methods of counterfactual analysis that can elevate your marketing efforts:
- Incrementality Testing
- Using Machine Learning Methods for Counterfactual Analysis
What is Incrementality Testing
Incrementality Testing is a robust method used to determine the true impact of a marketing activity by measuring the additional value it generates beyond what would have occurred without the intervention. Essentially, it helps answer the critical question: Did this marketing effort drive extra conversions, or would they have happened regardless?
How Does It Work
The core of Incrementality Testing lies in conducting controlled experiments or effectively isolating test and control groups in a statistically consistent manner. Here's a step-by-step overview:
- Define Objectives: Clearly articulate what you aim to measure, such as lead generation, conversion rates, or revenue growth.
- Segment Your Audience: Randomly divide your target audience into two groups—the test group (exposed to the marketing activity) and the control group (not exposed).
- Execute the Campaign: Implement your marketing activity (e.g., new campaign, creative content, messaging) targeting only the test group while keeping the control group unexposed.
- Collect and Analyze Data: Gather data on key performance indicators (KPIs) from both groups during and after the campaign period.
- Measure Incrementality: Compare the outcomes between the test and control groups to isolate the incremental impact of the marketing activity.
Benefits of Incrementality Testing
- Accurate Impact Measurement: Provides a clear understanding of the true effectiveness of your marketing efforts by isolating their direct impact.
- Actionable Insights: Offers actionable insights into which strategies are driving growth and which need refinement.
What is Machine Learning-Based Counterfactual Analysis
Machine Learning (ML) methods for counterfactual analysis leverage advanced algorithms to predict what would have happened in the absence of a specific marketing action. Unlike traditional methods, ML can handle vast amounts of data and uncover complex patterns that might be missed otherwise, providing a more nuanced understanding of marketing impacts.
How Does It Work
Machine Learning models are trained on historical data to understand the relationships between various marketing activities and outcomes. By simulating different scenarios, these models generate counterfactuals—estimations of what would have occurred without the intervention. This approach eliminates the need for running controlled experiments, making it a versatile tool for marketers.
Key ML Techniques for Counterfactual Analysis
- Markov Chains for Multi-Touch Attribution:
- Application: Helps in attributing conversions to multiple touchpoints in the customer journey.
- Benefit: Provides a probabilistic understanding of how different marketing interactions contribute to conversions, allowing for more accurate attribution.
- Multivariate Regression-Based Methods (Marketing Mix Analysis):
- Application: Assesses the impact of various marketing channels and tactics on overall performance.
- Benefit: Enables marketers to quantify the effectiveness of different marketing components and optimize their mix for maximum impact.
Different ML Methods for Different Decision Levels
Different ML methods serve various decision-making needs within marketing:
Marketing Mix Analysis (MMx) as Your Strategic Compass:
- Use Case: Ideal for channel-level decisions over the upcoming months and quarters.
- Application: Whether allocating budgets to Google, LinkedIn, Meta, or Conferences, MMx helps answer pivotal questions: How should spending be allocated across channels to maximize ROI and pipeline?
- Strength: Excels at guiding planning, budgeting, and allocation decisions. Ability to account for dark social interactions
- Limitation: Falls short in evaluating the effectiveness of specific campaign tactics and creatives or understanding the sequence of touches needed for funnel movements and conversions.
Probabilistic and Data-Based Multi-Touch Attribution (MTA):
- Use Case: Perfect for evaluating specific campaign tactics and daily or weekly tactical executions.
- Application: Facilitates decisions on reallocating resources and managing the funnel on a day-to-day basis.
- Strength: Captures impressions from digital channels, tracks anonymous visits, and follows the visitor's journey to conversion through the deep funnel.
- Benefit: Enhances the utility of attribution methods by understanding the sequence and impact of various touchpoints.
Advantages of Machine Learning Methods
- Scalability: Capable of processing and analyzing large datasets, making it ideal for complex B2B environments.
- Precision: Enhanced ability to identify nuanced patterns and interactions between variables, leading to more accurate counterfactuals.
- Automation: Streamlines the analysis process, reducing the time and effort required compared to manual methods.
Choosing the Right Method for Your Business
Both Incrementality Testing and Machine Learning-Based Counterfactual Analysis offer unique advantages for B2B marketers seeking to understand the true impact of their campaigns. The choice between them depends on various factors:
Data Availability:
- Machine Learning Methods: Require extensive and high-quality data.
- Incrementality Testing: Can be effective in low /no data scenarios
Resource Allocation:
- Machine Learning-Based Approaches: These may require specialized skills and computational resources.
- Incrementality Testing: This can be executed with standard marketing tools.
Business Objectives:
- Incrementality Testing: Ideal for straightforward lift measurement.
- Machine Learning Methods: Provide deeper analysis for more complex, multi-faceted insights.
Situations Where Each Method Excels
- Marketing Mix Analysis and Multi-Touch Attribution:
- Best For: Scenarios where historical data is available.
- Use Cases: Channel allocation, budget planning, understanding touchpoint contributions.
- A/B Testing and Incrementality Testing:
- Best For: Situations where data is limited or unavailable.
- Use Cases: Launching new ad creatives, testing new messages, expanding into new channels, and assessing the impact of one-time events.
Conclusion
Counterfactual analysis is an invaluable tool in the B2B marketer’s arsenal, enabling the precise measurement of campaign effectiveness and the optimization of marketing strategies. Whether through Incrementality Testing or Machine Learning-Based Counterfactual Analysis, understanding what truly drives your marketing success is essential for sustained growth and competitive advantage.
Ready to Elevate Your Marketing Strategy?
Implementing robust counterfactual analysis methods can transform your approach to B2B marketing, ensuring that every effort is backed by data-driven insights. Connect with us to discover how our solutions can help you harness the power of counterfactual thinking and drive meaningful business outcomes.
Check out our Future of B2B Attribution ebook to learn more.
